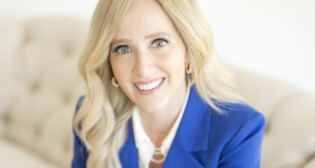
Which Intelligence, When?
Written by Ron Lindsey, Contributing Editor
RAILWAY AGE, SEPTEMBER 2023 ISSUE: For this article, Artificial Intelligence (AI), including Machine Learning (ML), is defined as the ability to perform tasks that are normally beyond the ability of human intelligence, either due to complexity, the amount of data, a time restriction, and/or continuous variation in the values of the variables involved. To be clear, ML is only one form of AI.
The opportunities for the use of AI involving train movements are not the same across the globe. For example, the applications of AI for train movements on North American railroads differ significantly from those of Europe and Asia. The two primary opportunities to be considered here are train control and traffic management that provide for increased safety and business benefits, respectively.
Train Control
For European railroads, the integration of AI with fiber-optic sensing (FOS) promises precise train detection and position leading to the digitization and automation of operations. However, in the U.S., the freight railroads already have very accurate train positioning (18 cm with 10-9 confidence level) and speed available via the PTC (Positive Train Control) platform where deployed.
As to the use of AI in train control in the U.S., PTC provides that for enforcement in both signaled and dark territories. PTC continuously evaluates the braking curves for multiple targets to ensure that an enforcement can be made if the engineer’s handling is predicted by the PTC platform to violate the parameters of the train’s movement authority. Targets include movement authority limits, speed restrictions, misaligned switches, and lack of permission to pass through a work zone.
Contrarily, ML is being implemented for enhanced safety beyond that of enforcement. I refer to 4AI Systems’ HORUS, an ML vision system to detect and classify objects in the rail corridor in real time. Its in-cab screen provides a forward view to the engineer with specific objects outlined and identified. This includes intrusions by people within rail danger zones.
Additionally, if so configured, HORUS can include braking for detected hazards. In time, the system can create a database of the wayside sufficient for block occupancy in lieu of track circuits. However, as with Virtual CTC (VCTC) that my consultancy designed for the railroads of Egypt and Kazakhstan to eliminate track circuits, this will not be implemented in North America any time soon. I say this due to the resistance to the resulting reduction in labor, as well as the reduction in revenue for suppliers.
Traffic Management
For the business end of rail operations, is use of AI practical? My perspective is based on Operations Research (OR) techniques stemming from my quantitative MBA education. OR consists of various mathematical techniques including Linear Programming (LP), Monte Carlo simulation, regression analysis, econometric modeling, etc. When one considers moving-block, arguably the most efficient traffic management approach for track time usage, the underlying process is the aggressive use of algebra, not unlike what many of us experienced in high school.
For example: Two trains are progressing toward each other at a certain time and speed. With the distance between the two locations known, one can make a deterministic calculation as to the meeting point and time using algebra. However, if other variables are considered—e.g., priority, customer value or schedule requirements—then the math becomes more complex, and algebra is not sufficient. Instead, LP is ideal for these types of problems that have constraints to be considered. However, LP is not meant for a continuous “live” analysis. What to do?
Throughout my professional career, I have often resorted to use of the heuristic 80/20 rule of achieving results. My designs of PTC and VCTC were partially done in that fashion, as well as various models and simulations I have performed. Simply stated, 80% of the objective can be completed with 20% of the effort that would be required for an absolute solution. This means addressing only a few major variables and using “in-time,” not real-time, positioning and speed data. It also means being able to make sound assumptions about some variables that are not really required for a reasonable solution. One OR tool I have used is Monte Carlo simulation. This process predicts possible outcomes of an uncertain event via many iterations that address the distribution of values for the key variables.
Hence, my bottom line for the use of AI to address various constraints of traffic management is that it is not practical for execution purposes. However, it can be of value for planning purposes.
Moving beyond use of AI for safety and business value in train movement handling, I address several areas below I know are in service or actively being pursued. Clearly, this list is not complete. The most common uses of AI in railroads fall into two general categories: ML and Optimization. I also describe one example of econometric modeling.
Machine Learning
ML is use of algorithms and statistical models to draw inferences from patterns in data.
BROKEN RAIL PROTECTION: Considering FOS again, ML is useful for broken rail protection where track circuits are not used—i.e., dark territory. This sophisticated capability is in revenue service in Europe, at least.
PREDICTIVE MAINTENANCE: Without any personal experience, I do know that ML is being used for predictive maintenance for various parts of the physical plant, including wayside infrastructure and rolling stock.
DYNAMIC HOSTLER ASSIGNMENTS: The availability of timely activity of units to be handled and train availability can minimize wasted travel time of the hostlers outside of the yard.
Optimization
Optimization analysis is used to minimize or maximize the value of a set of linear (most common) functions.
FUEL PURCHASING MANAGEMENT: In my early days at a Class I railroad, I developed LP that was used monthly to consider the spot prices of fuel at various locations and then to calculate the minimum total cost for buying, storing and transporting fuel to depots across the system. But a particular application of LP has to be understood and manageable. Second, management needs to buy in.
HOSTLER EFFICIENCY: Optimizing the distance and location of their activities can minimize the loading time in yards by hostlers.
INTERMODAL TRAIN ETA: By monitoring the dwell times of recent trains, the estimated time arrival of an intermodal train can be improved as to accuracy.
INTERMODAL LOADING OPTIMIZATION: The rules of the loading plan for containers and trailers can be quickly handled by ML.
Econometric Modeling
A second tier of AI possible for fuel purchase management is use of econometric modeling to predict the changes in fuel price in a one- to two-month time frame. I include this one based on what I was able to do in predicting scrap steel prices for a steel company I worked for prior to the railroads. It was very successful for deciding when to buy extra scrap and “lay it down.” Granted, as we all have experienced, there seems to be no justification in the swing of gasoline prices. I am not sure that is as true for diesel fuel.
In closing, AI should not be considered as something superficial or exotic. Nor should the term be used inappropriately for using human intelligence more effectively. Rather, it refers to the use of mathematics applied to the data available to develop a “best” solution where a deterministic solution cannot be achieved.